What is Data Science
- Wikioedia
- May 1, 2015
- 2 min read
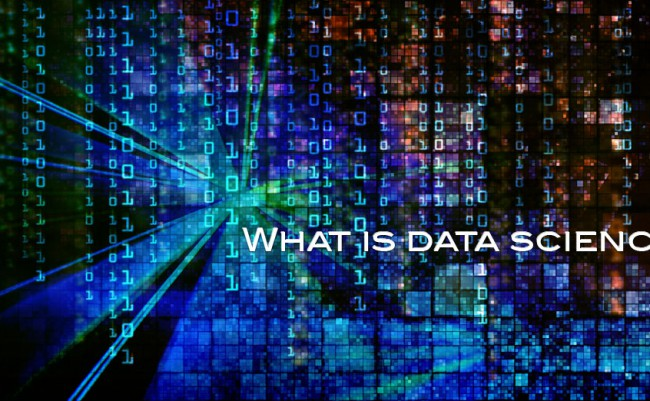
Data Science is an interdisciplinary field about processes and systems to extract knowledge or insights from large volumes of data in various forms, either structured or unstructured which is a continuation of some of the data analysis fields such as statistics, data mining and predictive analytics, as well as Knowledge Discovery in Databases (KDD).
Data science employs techniques and theories drawn from many fields within the broad areas of:
mathematics,
statistics,
chemometrics,
information science,
computer science,
signal processing,
probability models,
machine learning,
statistical learning,
data mining,
database,
data engineering,
pattern recognition and learning,
visualization,
predictive analytics,
uncertainty modeling,
data warehousing,
data compression,
computer programming, and
artificial intelligence.
Methods that scale to Big Data are of particular interest in data science, although the discipline is not generally considered to be restricted to such big data. The development of machine learning has enhanced the growth and importance of data science. At BIO Time Series we are appling data science approaches such as machine learning to create a predictive model that can learn based on current and past personalized biomarker time series, known disease data, and contextual factors, such as date, time, diet, medication etc. A future disease or health behavior can be output by the BIO time series based model using the personal biomedical baseline for a given biomarker or a panel of biomarkers and existing contextual factors for the individual.
Data science utilizes data preparation, statistics, predictive modeling and machine learning to investigate problems in various domains such as agriculture, marketing optimization, fraud detection, risk management, marketing analytics, public policy, and more recently in health. It emphasizes the use of general methods such as machine learning that apply without changes to multiple domains. This approach differs from traditional statistics with its emphasis on domain-specific knowledge and solutions. (The rationale is that developing tailored solutions does not scale.)
Data scientists use their data and analytical ability to find and interpret rich data sources; manage large amounts of data despite hardware, software, and bandwidth constraints; merge data sources; ensure consistency of datasets; create visualizations to aid in understanding data; build mathematical models using the data; and present and communicate the data insights/findings. They are often expected to produce answers in days rather than months, work by exploratory analysis and rapid iteration, and to get/present results with dashboards (displays of current values) rather than papers/reports, as statisticians normally do.
Comentários